Searching for in-vitro drug metabolites in LC-MS chromatograms without the aid of radiolabels
Peter L. Jacobs, Joop C. M. Waterval, N.V. Organon, Oss, The Netherlands
[This publication was originally presented as a poster at ASMS 2005. Reproduced here with permission.]
Overview
Novel Aspect: Pro’s and con’s of various software approaches used to search for in-vitro drug metabolites in early ADME
Introduction
In the classical metabolite identification approach one or more radiolabels are used to locate metabolites in lc(-ms) chromatograms and/or to isolate individual metabolites for off-line structure elucidation using MS, NMR and/or IR. The fast drug design cycles in early drug discovery do not allow synthesis of labelled drugs to support metabolite identification. Furthermore biotransformation sites have to be identified much more routinely e.g. to improve metabolic stability in new chemical series during lead optimization. Even with in-vitro incubations (microsomes, hepatocytes) the matrices used contribute to a large extend to the ion current observed and often mask metabolites formed. Various software approaches that have been used to speed-up metabolite identification in lead optimization within Organon will be summarized with a focus on low resolution applications and using Buspirone as a non-proprietary example.
Methods
The metabolism of Buspirone (5-HT Agonist, Antianxiety) is well documented (refs 1-3) and the compound has also featured in many application notes on metabolite identification. The short half-life in liver microsomes (rat ~2 min., human ~3.5 min.) and the large number of easy to analyse metabolites observed made us inject the acetonitril diluted/quenched samples (0.25 ?M) of a 1 ?M human liver microsomal incubation. For routine analysis in our drug discovery programs we obtain 10-100x less signal and therefore most in-vitro incubations for metabolite ID are both performed at 1 and 10 ?M.
Spectra were recorded with the Thermo LCQ Deca XP Plus ion trap and the ABI MDS Sciex API 4000 / 4000 QTrap.
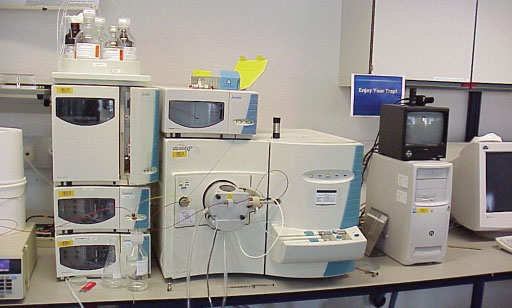
Figure 3. Thermo LCQ Deca XP Plus
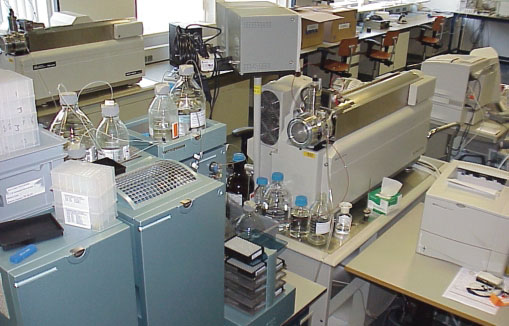
Figure 4. ABI MDS Sciex API 4000 / 4000 Qtrap
Various software packages have been used to search for metabolites in samples from in-vitro metabolic stability screens. These metabolite ID packages were either ms vendor specific (Thermo Metabolite ID 2.0, Bruker Metabolite Tools 1.1, ABI MDS Sciex Metabolite ID 1.2, Waters MetaboLynx 4.0), commercially available from other sources (e.g. ACD MS Manager 8.0, Sierra Analytics Apex 1.0, Novatia MetLab 1.03) or developed in-house (Matlab 4.2 based).
In our early ADME support this type of software is used to:
- search for unknowns
- XIC browsing
- search for the expected
- XIC’s using biotransformation lists
- search for similarity
- isotope pattern searching
- grouping metabolites on precursor ions or neutral losses
- product ion spectra comparison
- search for the known or predicted
- literature, metabolite databases, in-house project knowledge
Results
Searching for the unknowns: XIC browsing
In-house developed software (Matlab V4.2c.1)
This software was developed at Organon to manually inspect all XIC chromatograms of lcms runs of both stressed and control samples, with user defined XIC steps. Up to 6 lcms chromatograms can be browsed simultaneously. Via conversion to the NetCDF format, data from all MS vendors can be imported. In our experience visual comparison of lcms chromatograms obtained from stability studies with various formulations is helpful to minimize the risk impurities are missed. Therefore this approach was also used for in-vitro metabolite ID, however, this time, lcms chromatograms were compared of samples with different incubation times.
Browsing through all XIC’s from several lcms chromatograms is a comprehensive and sensitive approach (Figure 5 and 6). No prior threshold values are required. It is the user who selects the relevant XIC’s, those who show clear chromatographic peaks increasing with incubation time. The necessary human interaction is at the same time also the drawback of the method, preventing batch processing.
Searching for the expected: XIC’s using biotransformation lists
The use of biotransformation lists to facilitate reporting is a common feature of any ms vendor’s Metabolite ID package. The same is true for background subtraction of control incubation samples. Also many different algorithms are used for peak finding. In our hands mainly useful to locate major metabolites in in-vitro incubates. Metabolic labile compounds in (our) lead optimization projects however tend to produce many low intensity biotransformation products especially in the first drug design cycles. A similar problem is faced when compounds become stable towards the end of the design cycles and minor metabolites have to be searched e.g. as candidates for screening in subsequent PK studies to aid in the EDC selection process.
Thermo Xcalibur Metabolite ID
Searching for similarity: msms spectral comparison
Novatia Metlab Profiler 1.03
Searching for the known or predicted: structure sets
Sierra AnalyticsApex
The Apex software can be used to search for compounds using a set of structures e.g. those of known or predicted metabolites. The software calculates and searches for mass spectral characteristics (isotope patterns, fragments). In the example shown Buspirone and 8 potential metabolite structures were selected from the MDL Metabolite database. The human liver microsomal incubation samples were searched for their presence.
MDL Metabolite literature database 2002.2
Metabolite Prediction
Sets of predicted metabolite structures can be generated with e.g. Meteor (LHASA Ltd), MetabolExpert (CompuDrug) or MetabolitePredict (Bruker). The Apex software can again be used to search in the ms datafiles for calculated spectral characteristics of these structures. Fragment ions predicted, and found in available msms spectra, might also point to novel metabolites even when their exact structure was not in the predicted set. Therefore overprediction of metabolites, in combination with an Apex search, is the preferred approach in research applications. MetabolExpert (Pallas 3.1.1.2) predicted the smallest set of structures for Buspirone (11 of which none with a +32 Da mass difference).
Increasing the performance
Any search for metabolites will benefit from increased chromatographic resolution (peak capacity). Capillary lc with a trapping column and column switching enables injection of ‘larger’ volumes. A more practical approach to increase performance might be to use the Waters Acquity uplc technique or hplc with < 2 ?m packing material. Increasing the mass resolution and mass accuracy allows the subsequent use of the so-called ‘Mass Defect Filter’ to locate metabolites that match with the accurate mass of the parent drug.
Conclusions
MS vendor specific metabolite ID software packages are routinely used in our lead optimization programs. In addition we showed the use of:
- XIC browsing to search for unknowns
- AutoShift™ correlation of product ion spectra to aid interpretation
- structure sets of potential metabolites as input for searches on calculated spectral characteristics
- improved chromatographic performance and increased mass resolution to facilitate metabolite ID data processing
Acknowledgements
The authors want to thank Peter van Houtum (Organon Research) and Suzanne de Goeij (trainee) for resp. the Buspirone LCQ and API 4000 experiments as well as Jose Castro-Perez (Waters, Manchester, UK) for the Q-TOF Premier results.
References
- Kerns, E. H.; Rourick, R. A.; Volk, K. J.; Lee, M. S.; J Chromatogr, B: Biomed Appl 1997, 698
- Jajoo, H. K.; Blair, I. A.; Klunk, L. J.; Mayol, R. F.; Xenobiotica, 1990, 20 (8), 779
- Stanley, S. M. R.; J Mass Spectrom, 2000, 35 (3), 402.